Overview
Overview • Related Work • Hardware • Description • Tools • Datasets
The Canadian Longterm Outdoor UAV Longterm Dataset (CLOUD) contains over 30 km of visual-inertial flight data collected at 3 different locations across Canada. Specifically, these locations include paths in Suffield, Montreal and the University of Toronto Institute for Aerospace Studies (UTIAS) in Toronto. In these experiments, a DJI M600 UAV with gimballed camera is flown to create a Teach run which is immediately followed by a Repeat run traversed in the reverse direction. For each path, we also include Google Earth images. Data is provided in human-readable text files. A set of Python tools is provided to work with the data. Teach and Repeat runs can be download individually as needed. Our data is well-suited for research in UAV visual localization including robustness to perspective change, lighting change and seasonal changes. Other potential use cases include satellite image UAV navigation, experience-based localization and simulatenous localization and mapping. See Related Work for more past uses.
Suffield
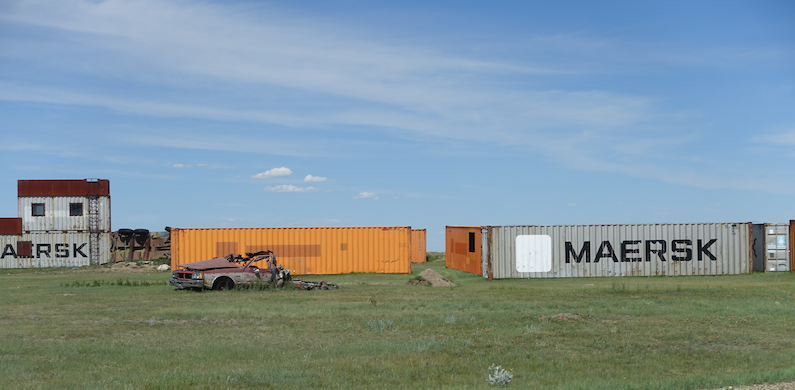
Montreal
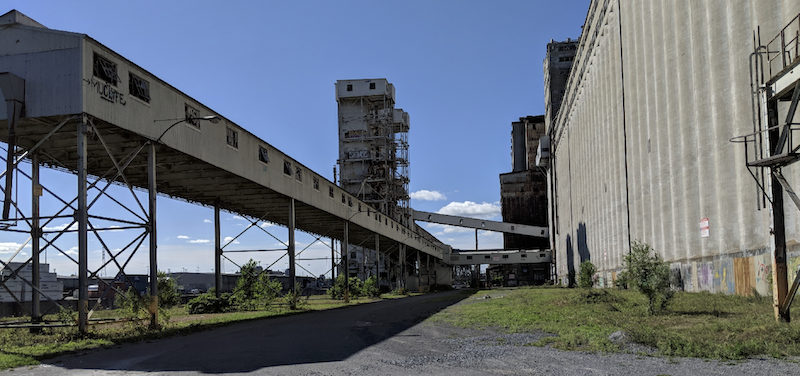
UTIAS
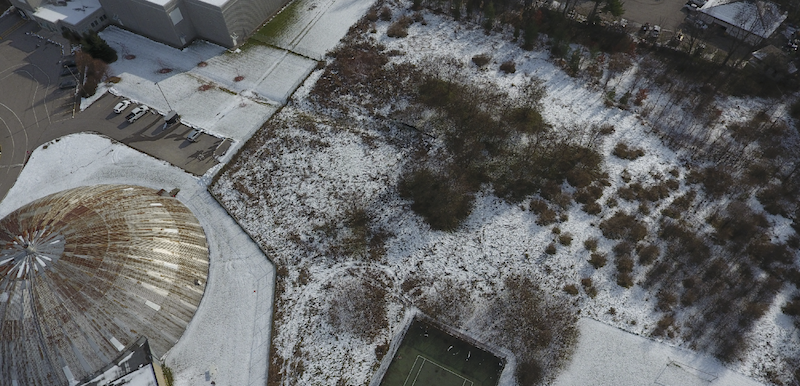
Related Work
CLOUD was collected from 2018-2020 in three distinct environments in Canada and across multiple seasons. To our knowledge, our dataset is currently the largest UAV visual outdoor dataset with over 30 Km of visual-inertial flight data. A key feature of our dataset is that we also provide satellite images (generated using Google Earth) along our various flown paths. Our data is often collected in a Teach and Repeat format (see [5]) where we fly a path (teach) and then reverse the path, often autonomously (repeat). We include multiple trials of a given path. Our data spans 3 distinct environments including both rural and urban locations.
EUROC [1] | UPenn Fast [2] | Zurich Urban [3] | Blackbird [4] | UZH-FPV Racing [5] | Ours | |
---|---|---|---|---|---|---|
Environments | 2 | 1 | 1 | 3 [Rendered] | 2 | 3 |
Location | Indoor | Outdoor | Outdoor | Indoor | Indoor/Outdoor | Outdoor |
Camera Rate (Hz) | 20 | 40 | 20 | 120 | 30/50 | 15 |
IMU Rate (Hz) | 200 | 200 | 10 | 100 | 500/1000 | 400 |
Top Speed (m/s) | 2.3 | 17.5 | 3.9 | 7.0 | 12.8/ 23.4 | 14.3 |
Satellite Images | No | No | Yes | No | No | Yes |
Total Flight (km) | 0.89 | 2.4 | 2 | ~ 60 | 6.17 | 36.58 |
[Bibtex]
@article{burri-ijrr16,
title={The {EuRoC} micro aerial vehicle datasets},
author={Michael Burri and Janosch Nikolic and Pascal Gohl and Thomas Schneider and Joern Rehder and Sammy Omari and Markus W Achtelik and Roland Siegwart},
journal={{The International Journal of Robotics Research}},
volume={35},
number={10},
pages={1157--1163},
year={2016}
}
Related Publications
The data included in CLOUD has been used in a number of publications on a variety of vision-based navigation topics:
![[View BibTeX]](https://www.dynsyslab.org/wp-content/plugins/../papercite-data/icons/icon-bib.gif)
![[View Abstract]](https://www.dynsyslab.org/wp-content/plugins/../papercite-data/icons/icon-cod.gif)
![[Download PDF]](https://www.dynsyslab.org/wp-content/plugins/../papercite-data/icons/icon-pdf.gif)
![[View Video]](https://www.dynsyslab.org/wp-content/plugins/../papercite-data/icons/icon-vid.gif)
We estimate the global pose of a multirotor UAV by visually localizing images captured during a flight with Google Earth images pre-rendered from known poses. We metrically localize real images with georeferenced rendered images using a dense mutual information technique to allow accurate global pose estimation in outdoor GPS-denied environments. We show the ability to consistently localize throughout a sunny summer day despite major lighting changes while demonstrating that a typical feature-based localizer struggles under the same conditions. Successful image registrations are used as measurements in a filtering framework to apply corrections to the pose estimated by a gimballed visual odometry pipeline. We achieve less than 1 metre and 1 degree RMSE on a 303 metre flight and less than 3 metres and 3 degrees RMSE on six 1132 metre flights as low as 36 metres above ground level conducted at different times of the day from sunrise to sunset.
@INPROCEEDINGS{patel-icra20,
title = {Visual Localization with {Google Earth} Images for Robust Global Pose Estimation of {UAV}s},
author = {Bhavit Patel and Timothy D. Barfoot and Angela P. Schoellig},
booktitle = {{Proc. of the IEEE International Conference on Robotics and Automation (ICRA)}},
year = {2020},
pages = {6491--6497},
urlvideo = {https://tiny.cc/GElocalization},
abstract = {We estimate the global pose of a multirotor UAV by visually localizing images captured during a flight with Google Earth images pre-rendered from known poses. We metrically localize real images with georeferenced rendered images using a dense mutual information technique to allow accurate global pose estimation in outdoor GPS-denied environments. We show the ability to consistently localize throughout a sunny summer day despite major lighting changes while demonstrating that a typical feature-based localizer struggles under the same conditions. Successful image registrations are used as measurements in a filtering framework to apply corrections to the pose estimated by a gimballed visual odometry pipeline. We achieve less than 1 metre and 1 degree RMSE on a 303 metre flight and less than 3 metres and 3 degrees RMSE on six 1132 metre flights as low as 36 metres above ground level conducted at different times of the day from sunrise to sunset.}
}
A perception-aware flatness-based model predictive controller for fast vision-based multirotor flightM. Greeff, T. D. Barfoot, and A. P. Schoelligin Proc. of the International Federation of Automatic Control (IFAC) World Congress, 2020, p. 9412–9419.
Despite the push toward fast, reliable vision-based multirotor flight, most vision- based navigation systems still rely on controllers that are perception-agnostic. Given that these controllers ignore their effect on the system’s localisation capabilities, they can produce an action that allows vision-based localisation (and consequently navigation) to fail. In this paper, we present a perception-aware flatness-based model predictive controller (MPC) that accounts for its effect on visual localisation. To achieve perception awareness, we first develop a simple geometric model that uses over 12 km of flight data from two different environments (urban and rural) to associate visual landmarks with a probability of being successfully matched. In order to ensure localisation, we integrate this model as a chance constraint in our MPC such that we are probabilistically guaranteed that the number of successfully matched visual landmarks exceeds a minimum threshold. We show how to simplify the chance constraint to a nonlinear, deterministic constraint on the position of the multirotor. With desired speeds of 10 m/s, we demonstrate in simulation (based on real-world perception data) how our proposed perception-aware MPC is able to achieve faster flight while guaranteeing localisation compared to similar perception-agnostic controllers. We illustrate how our perception-aware MPC adapts the path constraint along the path based on the perception model by accounting for camera orientation, path error and location of the visual landmarks. The result is that repeating the same geometric path but with the camera facing in opposite directions can lead to different optimal paths flown.
@INPROCEEDINGS{greeff-ifac20,
author = {Melissa Greeff and Timothy D. Barfoot and Angela P. Schoellig},
title = {A Perception-Aware Flatness-Based Model Predictive Controller for Fast Vision-Based Multirotor Flight},
booktitle = {{Proc. of the International Federation of Automatic Control (IFAC) World Congress}},
year = {2020},
volume = {53},
number = {2},
pages = {9412--9419},
urlvideo = {https://youtu.be/aBEce5aWfvk},
abstract = {Despite the push toward fast, reliable vision-based multirotor flight, most vision-
based navigation systems still rely on controllers that are perception-agnostic. Given that these controllers ignore their effect on the system’s localisation capabilities, they can produce an action that allows vision-based localisation (and consequently navigation) to fail. In this paper, we present a perception-aware flatness-based model predictive controller (MPC) that accounts for its effect on visual localisation. To achieve perception awareness, we first develop a simple geometric model that uses over 12 km of flight data from two different environments (urban and rural) to associate visual landmarks with a probability of being successfully matched. In order to ensure localisation, we integrate this model as a chance constraint in our MPC such that we are probabilistically guaranteed that the number of successfully matched visual landmarks exceeds a minimum threshold. We show how to simplify the chance constraint to a nonlinear, deterministic constraint on the position of the multirotor. With desired speeds of 10 m/s, we demonstrate in simulation (based on real-world perception data) how our proposed perception-aware MPC is able to achieve faster flight while guaranteeing localisation compared to similar perception-agnostic controllers. We illustrate how our perception-aware MPC adapts the path constraint along the path based on the perception model by accounting for camera orientation, path error and location of the visual landmarks. The result is that repeating the same geometric path but with the camera facing in opposite directions can lead to different optimal paths flown.},
}
There’s no place like home: visual teach and repeat for emergency return of multirotor UAVs during GPS failureM. Warren, M. Greeff, B. Patel, J. Collier, A. P. Schoellig, and T. D. BarfootIEEE Robotics and Automation Letters, vol. 4, iss. 1, p. 161–168, 2019.
Redundant navigation systems are critical for safe operation of UAVs in high-risk environments. Since most commercial UAVs almost wholly rely on GPS, jamming, interference and multi-pathing are real concerns that usually limit their operations to low-risk environments and VLOS. This paper presents a vision-based route-following system for the autonomous, safe return of UAVs under primary navigation failure such as GPS jamming. Using a Visual Teach and Repeat framework to build a visual map of the environment during an outbound flight, we show the autonomous return of the UAV by visually localising the live view to this map when a simulated GPS failure occurs, controlling the vehicle to follow the safe outbound path back to the launch point. Using gimbal-stabilised stereo vision alone, without reliance on external infrastructure or inertial sensing, Visual Odometry and localisation are achieved at altitudes of 5-25 m and flight speeds up to 55 km/h. We examine the performance of the visual localisation algorithm under a variety of conditions and also demonstrate closed-loop autonomy along a complicated 450 m path.
@article{warren-ral19,
title = {There's No Place Like Home: Visual Teach and Repeat for Emergency Return of Multirotor {UAV}s During {GPS} Failure},
author = {Michael Warren and Melissa Greeff and Bhavit Patel and Jack Collier and Angela P. Schoellig and Timothy D. Barfoot},
journal = {{IEEE Robotics and Automation Letters}},
year = {2019},
volume = {4},
number = {1},
pages = {161--168},
doi = {10.1109/LRA.2018.2883408},
urllink = {https://arxiv.org/abs/1809.05757},
urlvideo = {https://youtu.be/oJaQ4ZbvsFw},
abstract = {Redundant navigation systems are critical for safe operation of UAVs in high-risk environments. Since most commercial UAVs almost wholly rely on GPS, jamming, interference and multi-pathing are real concerns that usually limit their operations to low-risk environments and VLOS. This paper presents a vision-based route-following system for the autonomous, safe return of UAVs under primary navigation failure such as GPS jamming. Using a Visual Teach and Repeat framework to build a visual map of the environment during an outbound flight, we show the autonomous return of the UAV by visually localising the live view to this map when a simulated GPS failure occurs, controlling the vehicle to follow the safe outbound path back to the launch point. Using gimbal-stabilised stereo vision alone, without reliance on external infrastructure or inertial sensing, Visual Odometry and localisation are achieved at altitudes of 5-25 m and flight speeds up to 55 km/h. We examine the performance of the visual localisation algorithm under a variety of conditions and also demonstrate closed-loop autonomy along a complicated 450 m path.}
}
Hardware
The camera parameters are given here: camera_parameters.txt
The transformation (in the form of a vector followed by a quaternion) describing the rigid transform between the vehicle frame and the base frame is given here: from_base_to_vehicle.txt
The transformation (in the form of a vector followed by a quaternion) describing the rigid transform between the gimbal frame and the camera frame is given here: from_gimbal_to_camera.txt
The transformation (in the form of a vector followed by a quaternion) describing the transform between the gimbal frame (in neutral position – i.e. with the same orientation as the vehicle) and the vehicle frame is given here: from_vehicle_to_gimbal.txt
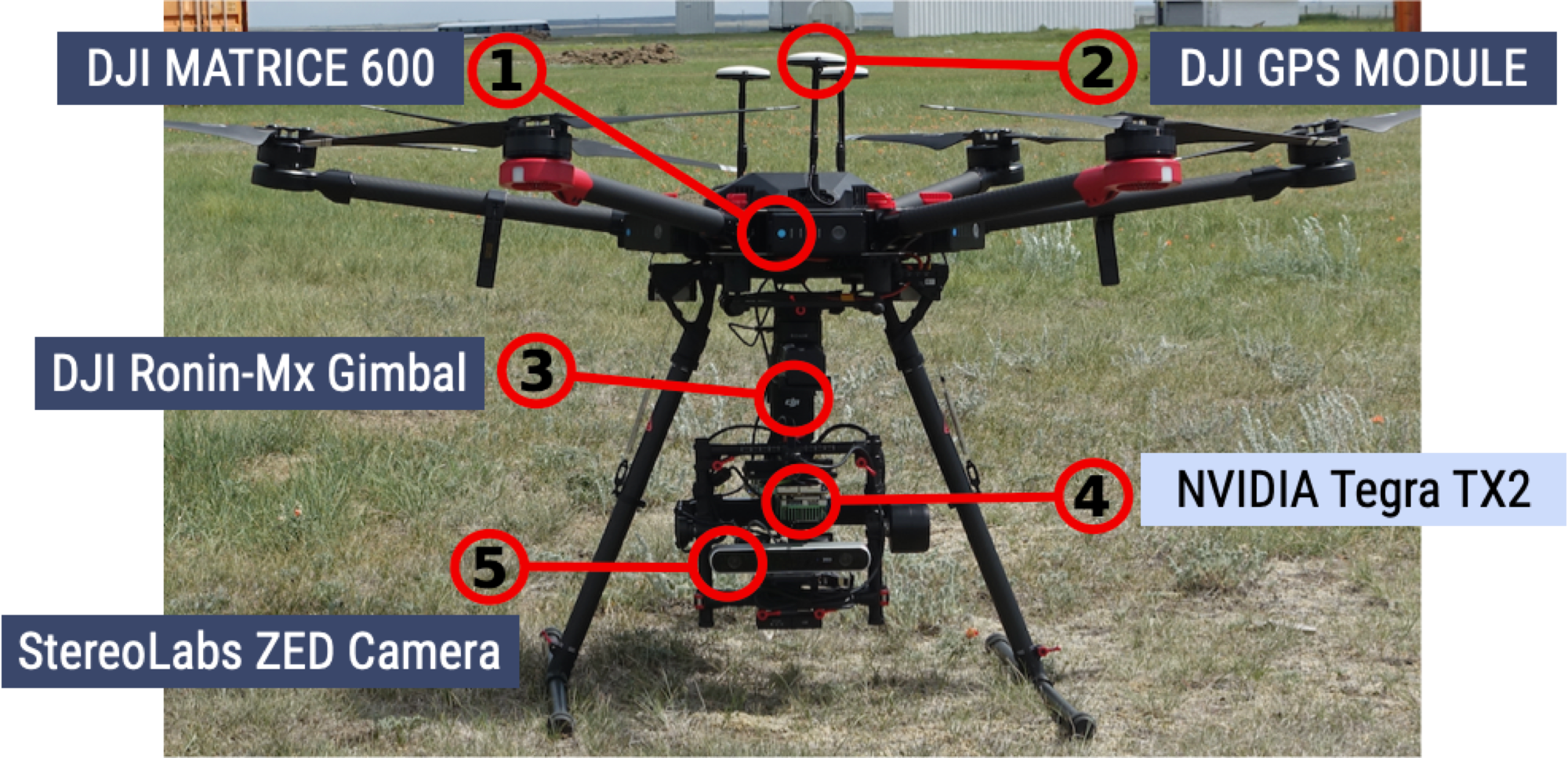
Description of Data Products
Coordinate Frames
The 4×4 homogeneous transformation matrices describing the rigid transformations between the sensor and vehicle frames are provided in the Hardware section.
Ground Frame: There are two Ground frames, i.e., East-North-Up (ENU) and North-East-Down (NED).
Vehicle and Base Frames: The Vehicle and Base frames are both Forward-Left-Up (FLU). The Vehicle frame is aligned at approximately the center-of-gravity of the M600 while the Base frame is shift down vertically as given in from_base_to_vehicle.txt.
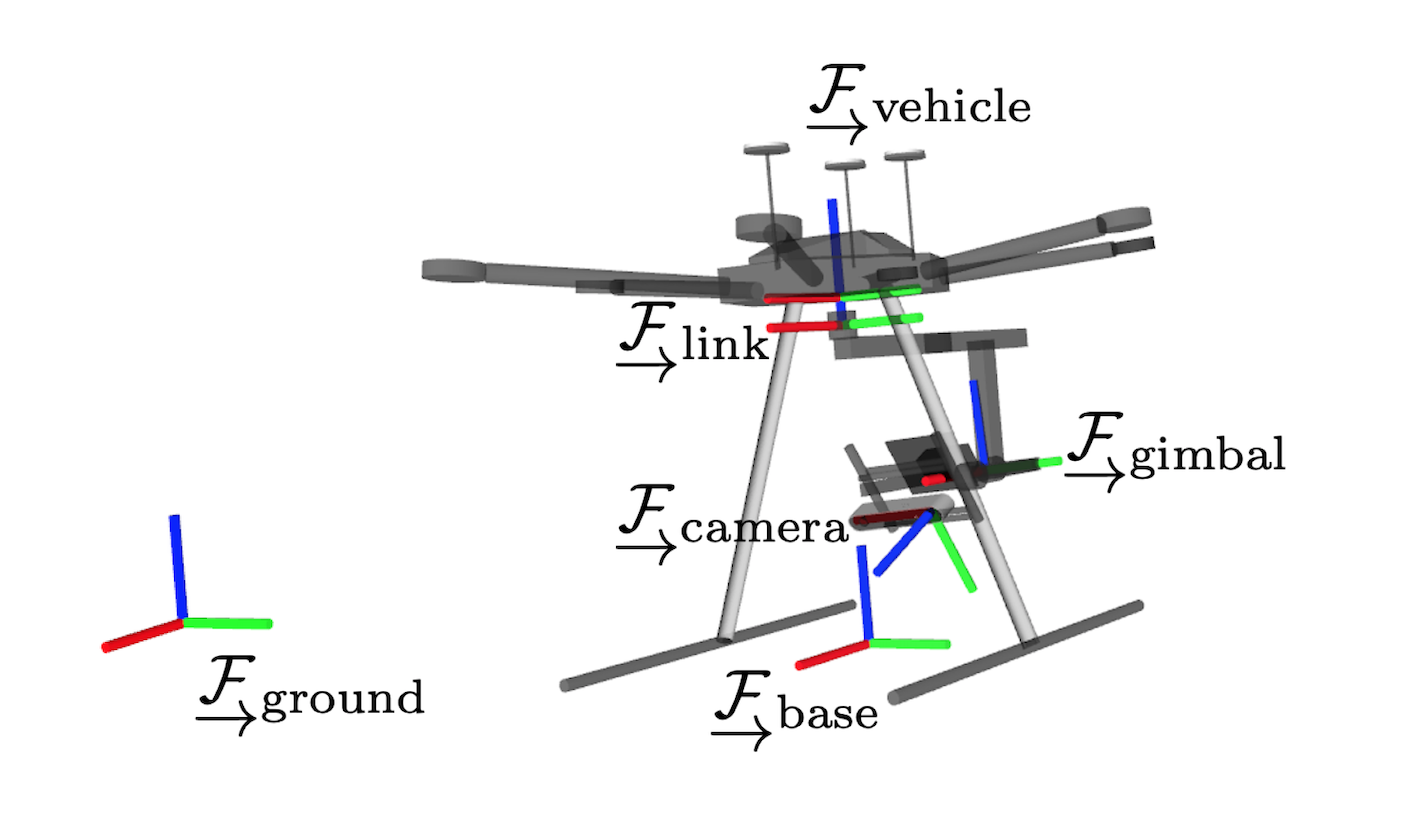
Stream |
Frame(s) |
Quantity |
Rate |
Raw/Estimated |
---|---|---|---|---|
velocities |
Ground |
Vector |
50 Hz |
Estimated |
attitude |
from Vehicle to Ground(ENU) |
Quaternion |
100 Hz |
Raw |
acceleration |
Vehicle |
Vector |
400 Hz |
Raw |
gps |
N/A |
GPS |
50 Hz |
Raw |
gimbal state |
from Base to Camera |
Vector, Quaternion |
50 Hz |
Estimated |
gimbal angle |
from Gimbal to Ground(NED) * |
Euler Angles |
50 Hz |
Raw |
image ids |
N/A |
Frame ID |
15 Hz |
Raw |
* The roll and pitch angles are given relative to the global NED frame, however the yaw angle is given relative to the Vehicle/Base frames. Unfortunately, this is the convention used in the DJI ROS SDK. We have provided a script to estimate the rotation (as a quaternion) from the Gimbal to the Vehicle/Base frames in the GitHub repository. However, for further convenience our estimated gimbal state (position and orientation) relative to the Base frame is also provided.
File Structure
The dataset is split into trial folders (which can be downloaded separately) each consisting of a teach and repeat run. Both teach and repeat runs contains an image subfolder with contain the images obtained from the left camera. Images are named in the order they were obtained. In each of the run folders the associated timestamp for each image can be found in image_id.txt. Alongside this, separate textfiles are provided with the GPS measurements, attitude, acceleration, velocities, gimbal angles and gimbal state obtained during either teach or repeat run.
Interpreting Text Files
All timestamps are Unix timestamps given in nanoseconds.
Vector Files
The acceleration, velocity and gimbal state data is stored as vector data. The vector data is contained in human-readable, comma-delimited text files. Each row provides the timestamp followed by the 3-D vector (x, y, z) in the associated frame given in the Description section.
Quaternion Files
The attitude and gimbal state data is stored as quaternion data. The quaternion data is contained in human-readable, comma-delimited text files. Each row provides the timestamp followed by the 4-D quaternion (x, y, z, w) which represents the rotation from frame A to frame B as listed in the Description section.
Frame ID Files
The images ids are also human-readable and comma-delimited with each row representing the timestamp followed by the associated image ID (as found in the images folder). The timestamp is a Unix timestamp in nanoseconds. These may be converted to UTC via standard datetime library functions.
GPS Files
The GPS measurement files are human-readable and comma-delimited with each row providing the timestamp, latitude, longitude and altitude (in m with respect to WGS 84 ellipsoid).
Euler Angles Files
Euler angles are in the standard ZYX convention (roll, pitch, yaw). They are given in degrees.
Helpful Tools
To aid in visualizing the data, some Python tools have been provided in a GitHub repository.
Dataset
This section provides individual zip files for each trial. A video preview is provided for each trial to quickly see weather and lighting conditions before downloading. To download multiple trials a script is provided that makes this easy: downloader.
Suffield
Trial | Teach | Teach Preview | Repeat | Repeat Preview | Download | ||||
---|---|---|---|---|---|---|---|---|---|
Start Time |
Max Speed |
Google Earth Images | Start Time |
Max Speed |
Google Earth Images | ||||
1 | 11:39 18-06-14 |
3.51 m/s | No | 11:41 18-06-14 |
4.43 m/s | No | |||
2 | 11:47 18-06-14 |
7.55 m/s | No | 11:49 18-06-14 |
7.64 m/s | No | |||
3 | 11:55 18-06-14 |
8.60 m/s | No | 11:57 18-06-14 |
8.69 m/s | No | |||
4 | 12:02 18-06-14 |
10.58 m/s | No | 12:04 18-06-14 |
10.68 m/s | No | |||
5 | 12:09 18-06-14 |
12.63 m/s | No | 12:11 18-06-14 |
12.16 m/s | No | |||
6 | 12:22 18-06-14 |
15.06 m/s | No | 12.23 18-06-14 |
13.27 m/s | No | |||
7 | 12:38 18-06-14 |
3.51 m/s | No | 12:41 18-06-14 |
3.43 m/s | No | |||
8 | 13:05 18-06-14 |
7.57 m/s | No | 13:07 18-06-14 |
7.56 m/s | No | |||
9 | 13:12 18-06-14 |
10.64 m/s | No | 13:14 18-06-14 |
10.81 m/s | No | |||
10 | 13:18 18-06-14 |
14.28 m/s | No | 13:20 18-06-14 |
10.62 m/s | No | |||
11 | 13:32 18-06-14 |
7.62 m/s | No | 13:34 18-06-14 |
7.70 m/s | No | |||
12 | 13:40 18-06-14 |
7.72 m/s | No | 13:42 18-06-14 |
7.62 m/s | No | |||
13 | 13:49 18-06-14 |
7.68 m/s | No | 13:51 18-06-14 |
7.62 m/s | No | |||
14 | 10:34 18-06-15 |
3.62 m/s | No | 10:37 18-06-15 |
4.07 m/s | No | |||
15 | 11:13 18-06-15 |
3.50 m/s | No | 11:15 18-06-15 |
3.82 m/s | No |
Montreal
Trial | Teach | Teach Preview | Repeat | Repeat Preview | Download | ||||
---|---|---|---|---|---|---|---|---|---|
Start Time |
Max Speed |
Google Earth Images | Start Time |
Max Speed |
Google Earth Images | ||||
1 | 14:07 18-09-11 |
2.47 m/s | Yes | 14:10 18-09-11 |
2.63 m/s | No | |||
2 | 14:22 18-09-11 |
3.47 m/s | No | 14:25 18-09-11 |
3.53 m/s | No | |||
3 | 14:39 18-09-11 |
3.52 m/s | No | 14:41 18-09-11 |
3.79 m/s | No | |||
4 | 16:18 18-09-11 |
3.52 m/s | No | 16:21 18-09-11 |
2.81 m/s | No | |||
5 | 16:32 18-09-11 |
3.60 m/s | Yes | 16:35 18-09-11 |
2.76 m/s | No | |||
6 | 16:46 18-09-11 |
3.61 m/s | No | 16:49 18-09-11 |
2.35 m/s | No | |||
7 | 10:11 18-09-10 |
2.43 m/s | No | 10:14 18-09-10 |
2.44 m/s | No | |||
8 | 12:47 18-09-10 |
2.59 m/s | No | 12:50 18-09-10 |
2.89 m/s | No | |||
9 | 15:16 18-09-10 |
2.63 m/s | No | 15:20 18-09-10 |
2.68 m/s | No | |||
10 | 16:48 18-09-09 |
2.56 m/s | No | 16:51 18-09-09 |
2.57 m/s | No |
UTIAS Field
Trial | Teach | Teach Preview | Repeat | Repeat Preview | Download | ||||
---|---|---|---|---|---|---|---|---|---|
Start Time |
Max Speed |
Google Earth Images | Start Time |
Max Speed |
Google Earth Images | ||||
1 | 11:16 19-08-16 |
5.60 m/s | 11:17 19-08-16 |
6.26 m/s | |||||
2 | 11:23 19-08-16 |
5.57 m/s | 11:24 19-08-16 |
6.93 m/s | |||||
3 | 11:35 19-08-16 |
5.63 m/s | 11:36 19-08-16 |
8.81 m/s | |||||
4 | 11:42 19-08-16 |
5.62 m/s | 11:43 19-08-16 |
10.84 m/s | |||||
5 | 11:44 19-08-16 |
5.62 m/s | 11:50 19-08-16 |
11.89 m/s |
UTIAS Circle
Trial | Teach | Teach Preview | Repeat | Repeat Preview | Download | ||||
---|---|---|---|---|---|---|---|---|---|
Start Time |
Max Speed |
Google Earth Images | Start Time |
Max Speed |
Google Earth Images | ||||
1 | 13:40 18-10-25 |
3.37 m/s | 11:42 18-10-26 |
3.71 m/s | |||||
15:30 18-10-26 |
3.55 m/s | ||||||||
09:25 18-10-29 |
4.31 m/s | ||||||||
16:42 18-10-30 |
3.62 m/s | ||||||||
17:52 18-10-30 |
4.08 m/s | ||||||||
13:46 18-11-05 |
3.79 m/s | ||||||||
16:20 18-11-08 |
4.56 m/s | ||||||||
12:10 18-11-12 |
3.64 m/s | ||||||||
2 | 14:37 18-11-08 |
3.49 m/s | 14:42 18-11-08 |
3.92 m/s | |||||
14:49 18-11-08 |
4.73 m/s | ||||||||
14:57 18-11-08 |
7.20 m/s | ||||||||
15:09 18-11-08 |
8.67 m/s |
UTIAS Straight
Trial | Teach | Teach Preview | Repeat | Repeat Preview | Download | ||||
---|---|---|---|---|---|---|---|---|---|
Start Time |
Max Speed |
Google Earth Images | Start Time |
Max Speed |
Google Earth Images | ||||
1 | 16:08 19-02-11 |
3.37 m/s | 16:27 19-02-11 |
3.38 m/s | |||||
16:30 19-02-11 |
3.24 m/s | ||||||||
16:34 19-02-11 |
3.36 m/s | ||||||||
16:41 19-02-11 |
3.35 m/s | ||||||||
16:45 19-02-11 |
3.40 m/s |
UTIAS Day
Trial | Teach | Teach Preview | Repeat | Repeat Preview | Download | ||||
---|---|---|---|---|---|---|---|---|---|
Start Time |
Max Speed |
Google Earth Images | Start Time |
Max Speed |
Google Earth Images | ||||
1 | 10:37 19-08-01 |
3.75 m/s | |||||||
2 | 11:56 19-08-01 |
3.79 m/s | |||||||
3 | 14:35 19-08-01 |
3.68 m/s | |||||||
4 | 17:50 19-08-01 |
3.61 m/s | |||||||
5 | 20:24 19-08-01 |
3.68 m/s |
UTIAS Winter
Trial | Teach | Teach Preview | Repeat | Repeat Preview | Download | ||||
---|---|---|---|---|---|---|---|---|---|
Start Time |
Max Speed |
Google Earth Images | Start Time |
Max Speed |
Google Earth Images | ||||
1 | 15:55 20-11-05 |
3.56 m/s | |||||||
2 | 15:49 20-11-16 |
3.53 m/s | |||||||
3 | 14:08 20-11-23 |
3.46 m/s | |||||||
4 | 11:58 20-12-10 |
3.5 m/s |
License
MIT License
Copyright (c) 2020 Autonomous Space Robotics Lab at the University of Toronto
Permission is hereby granted, free of charge, to any person obtaining a copy of this software and associated documentation files (the “Software”), to deal in the Software without restriction, including without limitation the rights to use, copy, modify, merge, publish, distribute, sublicense, and/or sell copies of the Software, and to permit persons to whom the Software is furnished to do so, subject to the following conditions:
The above copyright notice and this permission notice shall be included in all copies or substantial portions of the Software.
THE SOFTWARE IS PROVIDED “AS IS”, WITHOUT WARRANTY OF ANY KIND, EXPRESS OR IMPLIED, INCLUDING BUT NOT LIMITED TO THE WARRANTIES OF MERCHANTABILITY, FITNESS FOR A PARTICULAR PURPOSE AND NONINFRINGEMENT. IN NO EVENT SHALL THE AUTHORS OR COPYRIGHT HOLDERS BE LIABLE FOR ANY CLAIM, DAMAGES OR OTHER LIABILITY, WHETHER IN AN ACTION OF CONTRACT, TORT OR OTHERWISE, ARISING FROM, OUT OF OR IN CONNECTION WITH THE SOFTWARE OR THE USE OR OTHER DEALINGS IN THE SOFTWARE.